This post is part 2 of our series “The Future of Healthcare is Here: Arionkoder’s Comprehensive Guide to AI in Medicine.” Find part one here, and stay tuned for more insights and practical tips from Arionkoder’s experts on harnessing the power of artificial intelligence in healthcare.
In the first article of our series, we analyzed the impact that AI has made on the healthcare industry, and how this technology can be adopted to improve patients’ lives and make a difference.
Crafting solutions for problems in this domain is challenging due to the unique complexities of working with medical data. Factors such as data size and dimensionality, privacy and security, quality, integration, annotation, and interpretation make medicine a unique and demanding application field. Furthermore, projects in healthcare demand a high level of interdisciplinarity, as clinicians and machine learning experts need to interact with each other to determine the actual demands and the most effective and correct way to accomplish them. Last but not least, the fact that patients’ lives and health are at stake calls for a bulletproof engineering process that ultimately needs to guarantee an accurate, safe, and trustworthy solution.
At Arionkoder, we strongly believe that the best way to achieve that is by starting with the right foot. And, in our experience, the first fundamental step to achieve this is by capturing accurate and comprehensive requirements right at the outset. But, again, the peculiarities of this field call for a process much more complex than the one applied in standard software development.
In general, we try to map the problem presented by our healthcare clients in a two-dimensional space in which one is the nature of the data, and the other is the nature of the problem. Both dimensions are intertwined with one another, and this mapping process is not simple. The type and format of the data that will be used, jointly with its size and availability, filters out which kind of AI solutions can be applied. On the other hand, the nature of the problem also calls for specific models: if the target is to identify the presence/absence or grade of a certain condition, then the problem is treated as classification or as a ranking task; but if the objective is to predict a continuous value such as the amount of medication that should be administered to the patient, then we need to apply regression models.
To reduce the communication gap with our clients and make capturing requirements as smooth as possible, we usually offer them a series of training workshops in which we delve into the essentials of AI and the peculiarities of these two dimensions we referred to before. Let’s start today by analyzing the data dimension.
The data dimension
Clinical databases are multimodal, meaning they are filled with data acquired from multiple sources and devices and stored in different formats. The first question we ask our clients is which data will be applied for the required task. Once we understand how it is obtained and what its main characteristics are, we move forward to more complex details such as how it is stored, its availability and dimensionality, and the most cost-effective way to extract, analyze, and evaluate its utility for training machine learning algorithms.
Depending on our clients’ requirements, the input data can come in the following types:
- Electronic health records. This data includes patient demographics (age, gender, location, etc.), medical history, lab results, medications, and other clinical information. It is frequently stored in relational databases within hospitals’ systems, and machine learning algorithms to process it are frequently those designed for tabular data. These samples are used for tasks such as predicting patient outcomes, identifying risk factors for diseases, and providing decision support for clinicians.
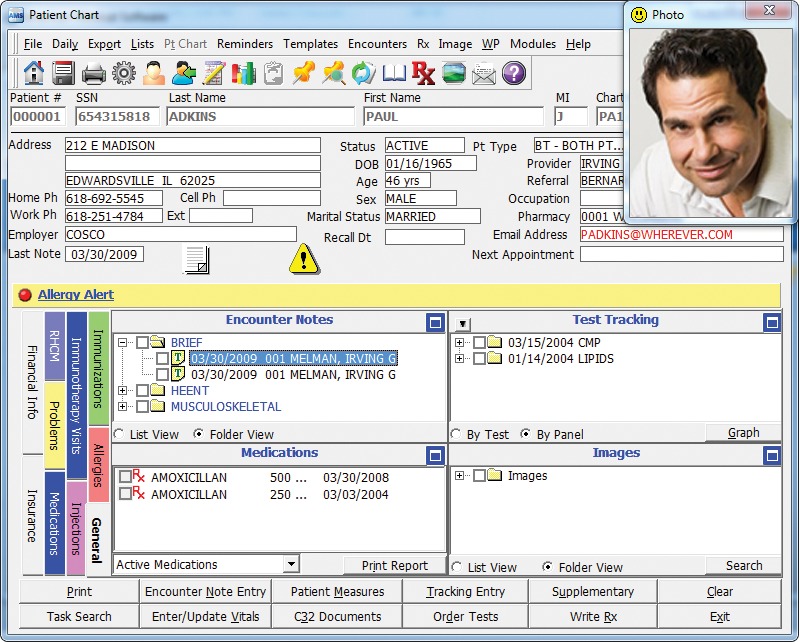
Source: https://www.emrsystems.net/american-medical-software/
Medical images. Medicine has been revolutionized by the multiplicity of imaging acquisition devices currently available. X-rays, CT scans, MRIs, OCTs, ultrasound images, etc., are used on a daily basis for clinicians and radiologists to provide diagnosis, plan treatments, and monitor diseases. Depending on the imaging modality, this data can be 2D or 3D and sometimes includes a time dimension to capture motion, such as Cine MRIs. Automatically processing this data is a subfield of machine learning known as Computer Vision, and is challenging in itself due to the complexity and dimensionality of images. Common applications of AI in medical imaging include detecting and classifying abnormalities, assisting radiologists with diagnosis, and quantifying features from organs or lesions, among others.
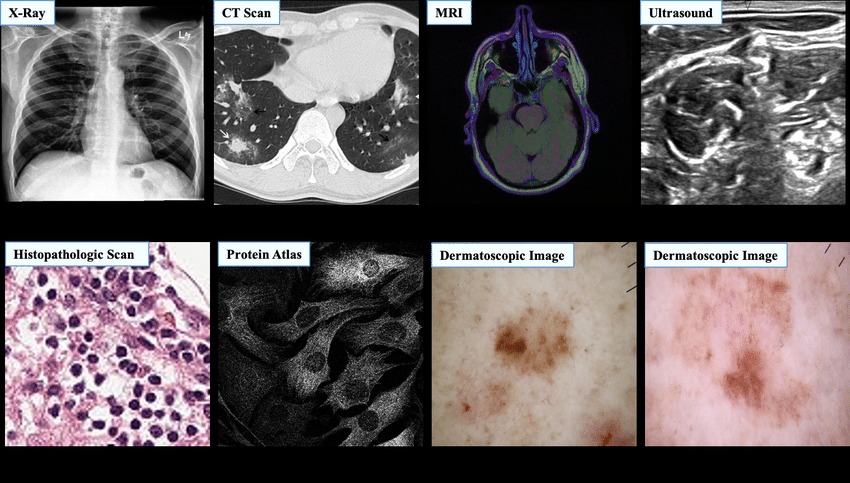
Source: http://dx.doi.org/10.3390/math8122192.
Genomic data. This data refers to the genetic information of an individual, including DNA sequences, variations, and mutations. Clinicians and researchers use this information to understand the underlying genetic causes of diseases and to develop personalized medicine strategies. Acquiring these samples is usually expensive, and processing them is challenging due to their high dimensionality and the low availability of samples. AI is being applied to genomic data to help with tasks such as identifying new drug targets, predicting patient response to treatments, and detecting genetic variations associated with diseases. It is also frequently used to analyze large-scale genomic data sets to identify patterns and associations that would be difficult to detect by manual means, e.g. for precision medicine applications. We’re already helping a company create their precision medicine and oncology solution! You can read all about it here.
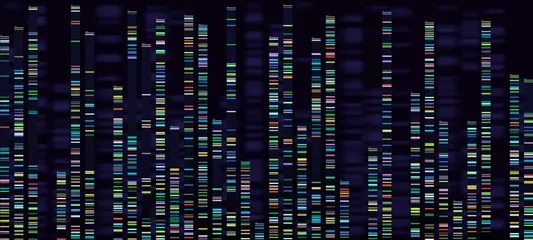
Source: https://geneticgenie.org/.
Biomedical signals. These refer to electrical, chemical, or mechanical signals produced by the body and measured either with clinical instruments–such as electrocardiograms (ECGs), electroencephalograms (EEGs), and electromyograms (EMGs)–, wearable devices–e.g. fitness trackers–or ICU sensors. These signals contain valuable information about patients’ physiological state and are used to monitor, diagnose, and treat a wide range of medical conditions. Machine learning models that exploit their time dimension and non-linearities are applied on this data to extract meaningful features, classify patterns, and make predictions. A few examples are detecting and diagnosing cardiac disorders such as arrhythmias from ECG, or detecting seizures in patients with epilepsy from EEG.
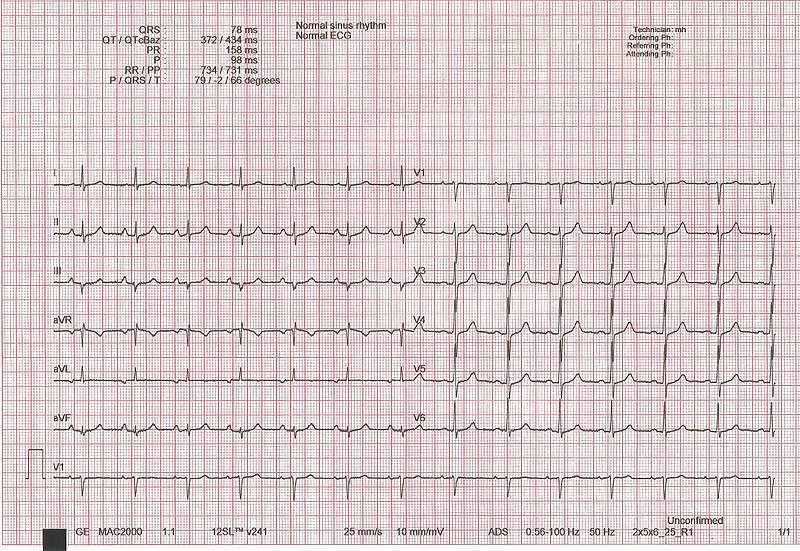
Source: https://www.lecturio.com/concepts/normal-electrocardiogram-ecg/
Clinical text. Electronic records usually include narrative information produced by clinicians as medical notes or discharge summaries. This data contains valuable information about patient history, diagnosis, treatment and outcomes, but it’s unstructured, highly dimensional, and tends to be full of medical jargon. Machine learning models for Natural Language Processing (NLP) such as those for named entity recognition, sentiment analysis and topic modeling are frequently applied to extract valuable information from this kind of text, such as predicting the likelihood of hospital readmission, or to identify patients at high risk of developing certain medical conditions.
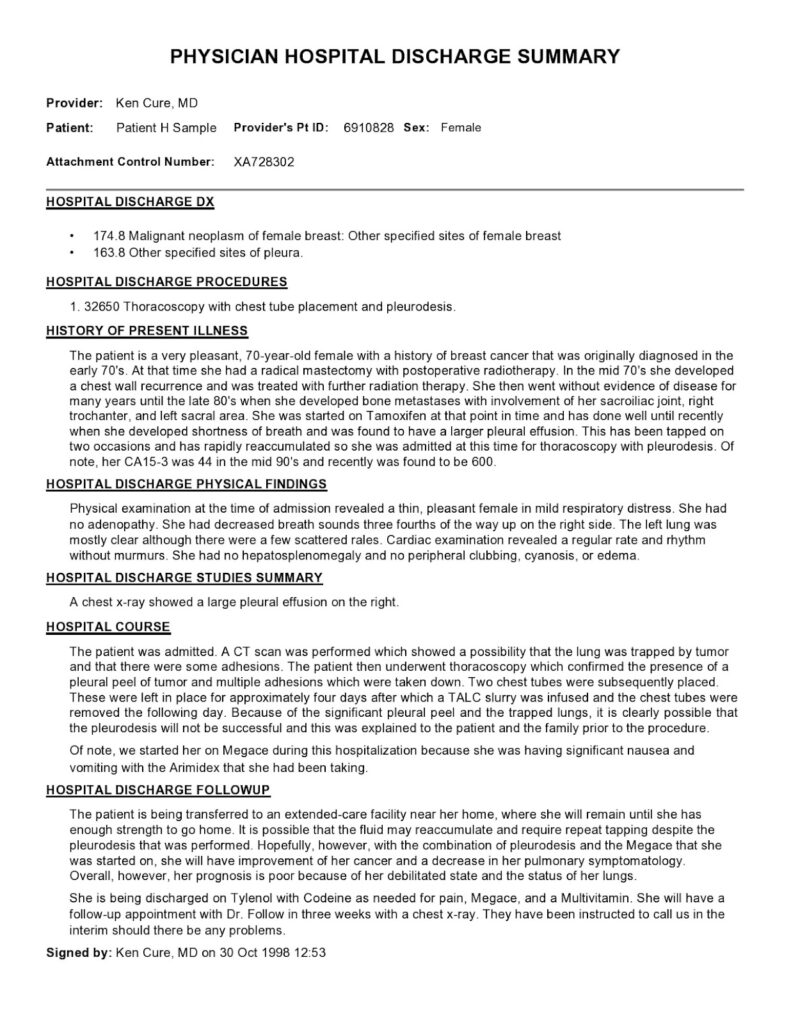
Source: https://templatearchive.com/discharge-summary/
Finally, one important factor in the data dimension is the fact that some applications call for analyzing and processing multimodal data and combine their intrinsic information. Imagine a computer vision problem such as disease detection from MRI, in which all the available scans are not explicitly labeled as diseased or not diseased, but are provided jointly with medical notes taken by a radiologist. By applying NLP techniques on these unstructured texts, we can predict whether its associated MRI comes from a diseased patient or not, and then use these labels for training a deep neural network classifier that solved the original problem.
In order to efficiently extract insights from these different types of data, understanding their characteristics and the best way to process them is essential. Our team at Arionkoder has extensive experience in working with different types of healthcare data, and we can help you identify the best data sources, extract and preprocess them, and apply state-of-the-art machine learning algorithms to extract insights and make predictions.
Stay tuned for our upcoming articles covering the problem dimension, and how we entwined both data and problems in Arionkoder to come up with the best possible AI tools.