By José Ignacio Orlando, PhD -Subject Matter Expert in AI/ML @ Arionkoder- and Nicolás Moreira -Head of Engineering @ Arionkoder-.
Part 1: Exploring the Basics of AI in Healthcare
Artificial intelligence (AI) models based on machine learning have made significant improvements in recent years, achieving remarkable results that have revolutionized many fields including medicine. In the healthcare industry, AI has enabled new diagnostic and treatment methods, improved patient care and outcomes, and eased the work of clinicians and healthcare providers.
One of the main reasons for the success of AI in medicine is the vast amount of data generated in the healthcare industry. Patient records, image scans, results of clinical studies and other types of data are constantly collected as part of standard medical processes. This data can be used to train machine learning models to automate tasks such as diagnosis and prognosis, treatment planning, drug design, and more.
Current medical AI solutions can process several types of clinical data. Electronic health records in the form of database tables can be analyzed using machine learning models to predict patient outcomes or identify specific diseases. Medical images, such as X-rays or MRIs, can be processed using deep learning to localize abnormalities and assist radiologists in diagnosis. Other signals such as EEG or ECG can be automatically analyzed with AI models to recognize patterns and predict potential health issues. In addition, AI can be used to process and analyze large amounts of text data, such as medical literature or patient notes, to extract relevant information and insights that could be ultimately exploited by clinical experts.
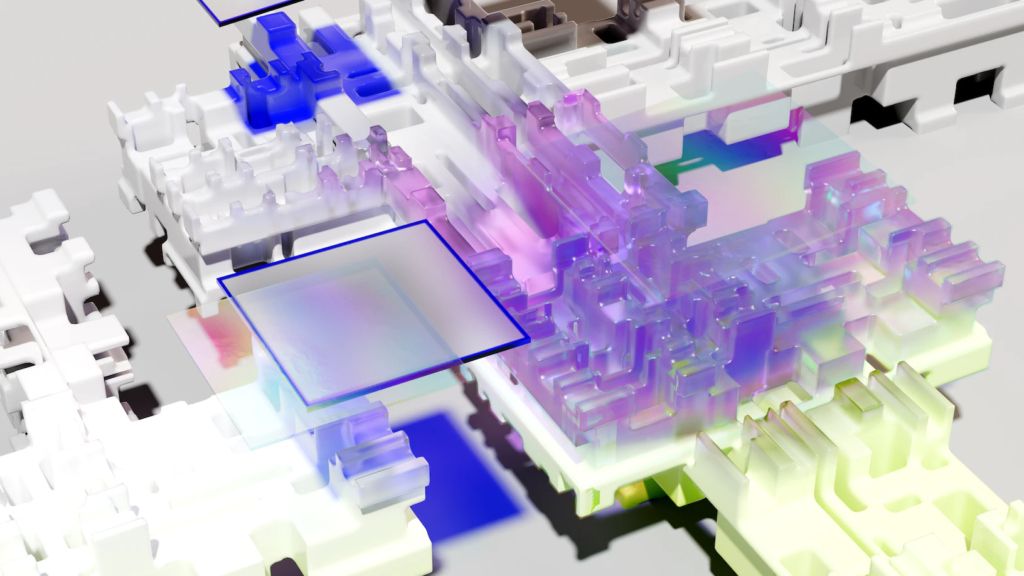
The advantages of implementing AI in clinical processes are multiple. Some of them are:
- Improved efficiency. AI models can analyze large databases to improve the efficiency of healthcare systems by automating tedious and time-consuming tasks that would be impractical to do manually. For example, AI algorithms are being used to delineate tumoral lesions in MRI, which is extremely valuable for planning effective radiotherapy treatments and assessing evolution over time. Instead of requiring a radiologist to “paint” the image voxel by voxel, convolutional neural networks can segment the areas of interest, discriminating normal tissue from tumoral edema, vascularized areas of the tumor, and necrotic tissue. This allows radiologists to use their time much more efficiently and focus on other critical tasks such as identifying subtle abnormalities, which is more challenging to automate.
- Enhanced accuracy. AI algorithms can be used as clinical support tools to assist experts in making faster and more accurate decisions. Automated disease diagnosis models, for example, can be used by doctors as a second opinion, and their outputs can be analyzed to improve diagnosis. Algorithms that automatically detect lesions can also help radiologists identify diseased areas that may be hidden in images due to their small size or lack of contrast, improving patient outcomes through more accurate diagnoses and access to appropriate treatments. This is especially important in the context of precision medicine, where large amounts of data must be integrated and analyzed to make personalized decisions.
- Increased accessibility. AI can bring healthcare decisions to remote or underserved areas where the availability of doctors is limited and the cost of bringing more of them is prohibitive. Machine learning-based models for automated disease detection, for instance, are currently being applied to efficiently screen for diseases in high-risk populations, identifying cases that require treatment and filtering out those that do not. This helps reduce the burden on clinicians, enabling them to focus on treating patients at risk and skipping healthy individuals. Furthermore, AI models that automatically classify the severity of a disease can be used for triaging and identifying which patients require more urgent medical attention, which allows for a much more efficient use of the existing resources.
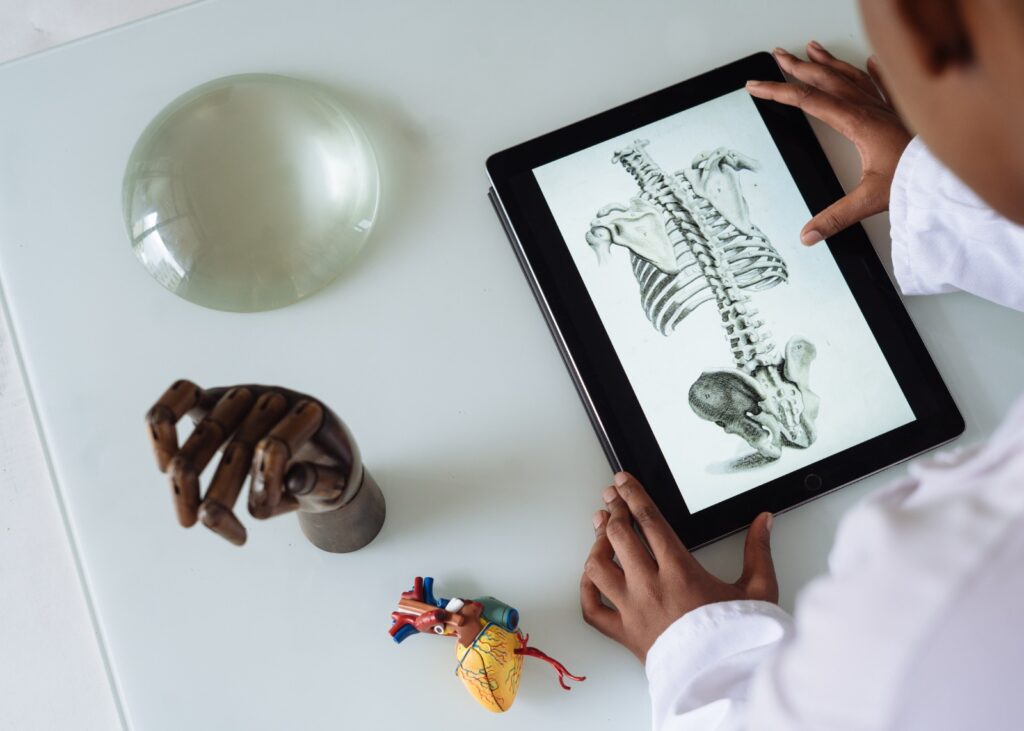
Despite the many potential benefits of AI in medicine, there are also several limitations and challenges that must be addressed in order to ensure the success of these tools:
- Data quality issues. One major challenge is the need for high-quality data to train and validate the models. In some cases, the data may be too scarce or too biased to generate accurate models, particularly in underserved or low-income areas. While collecting more diverse samples might sound like a quick optimal solution, what if their cost is prohibitive or if finding them is way too difficult? To overcome this limitation, it is necessary to take an interdisciplinary approach from the very beginning of the project, getting experts in data science and machine learning involved right from scratch and working closely with healthcare professionals responsible for data collection. This aids to ensure appropriate training sets for the ultimate goals and prevent dangerous dead-ends.
- Ensuring accuracy and reliability. To guarantee the success of the deployed AI solution, it is essential to make sure that the model is sufficiently robust to work accurately on new, unseen samples. This can be measured in advance by following appropriate evaluation processes on held-out test sets. Senior machine learning professionals can design rigorous protocols in collaboration with domain experts, and interact with them to identify potential pitfalls and delineate ways to mitigate them. Furthermore, systematically monitoring responses once the model is deployed is also important to prevent eventual failures due to drifts in data distribution.
- Lack of transparency of machine learning models. There is also a need to ensure the transparency and interpretability of AI models, particularly in the medical field, where lives may be at stake. The degree of interpretation is always related to the final application, so having experts involved in the design of the solution is extremely relevant to have a clear understanding of what will be the use–cases of the final system. Do clinicians want to see attribution maps highlighting areas in the images considered by the model to make a certain decision? In that case, which algorithms are the most appropriate to that end? Are uncertainty estimates needed too? Being able to ask these questions in advance usually requires having senior data scientists involved, as they need to know which kinds of tools are aligned with the requirements and how they can be produced.
- Preventing biases. In AI, bias refers to the systematic and unfair representation of certain groups or categories of people in the data and algorithms used to develop and deploy AI systems. In medicine, this can have serious consequences, as it can result in unequal and unfair treatment of patients, perpetuating existing inequalities in healthcare instead of solving them. For example, if an AI model for diagnosing a particular disease is trained on data that is predominantly from a certain race or ethnicity, it may be more accurate for that group but less accurate for others, leading to unequal and potentially harmful treatment for patients from underrepresented groups. Bias can also be introduced through the design and implementation of the models, for example if the model’s architecture or its training algorithms are biased towards certain groups or if the model is validated on biased data. Any AI project in medicine should implement robust ethical and regulatory frameworks, perform bias audits and assessments, and ensure transparency and accountability in the deployment and use of the final solution.
In the past decade, many companies and startups have started to successfully deliver AI solutions to improve daily healthcare practice. From clinical support systems that can automatically diagnose diseases to machine learning-driven tools that assist radiologists in analyzing medical images, AI is the present of medicine, and with no doubt its role will be critical in the future.
During the upcoming weeks, we will present Arionkoder’s vision on how to successfully employ AI for medical applications. We will do so with a series of articles covering each of what we believe are the building blocks to successfully design, implement, deploy and maintain reliable, trustworthy data-driven solutions to medicine. In this first episode of the series, we focused on the broader picture of the field. Stay tuned for the upcoming chapters, where we will dive into details regarding data quality, model design and evaluation and the technological infrastructure needed.
If your organization has a need for AI in medicine, at Arionkoder we’re ready to help you! Our team of experts is skilled in the latest AI technologies for healthcare applications, and we can assist you in implementing machine learning and deep learning solutions to improve the efficiency, accuracy, and cost-effectiveness of your operations. Reach out today to learn more about how we can support your AI projects!