Retrieval Augmented Generation (RAG) stands as one of the most influential techniques for extracting insights from vast databases. By simply posing a text-based query, these systems swiftly pinpoint relevant information sources and provide human-like responses instantly, sparing us the effort of constructing them manually.
In our ongoing series, we’ve explored various applications of this technology and delved into its operational fundamentals. In this new article, we aim to delve deeper into one pivotal component: the data.
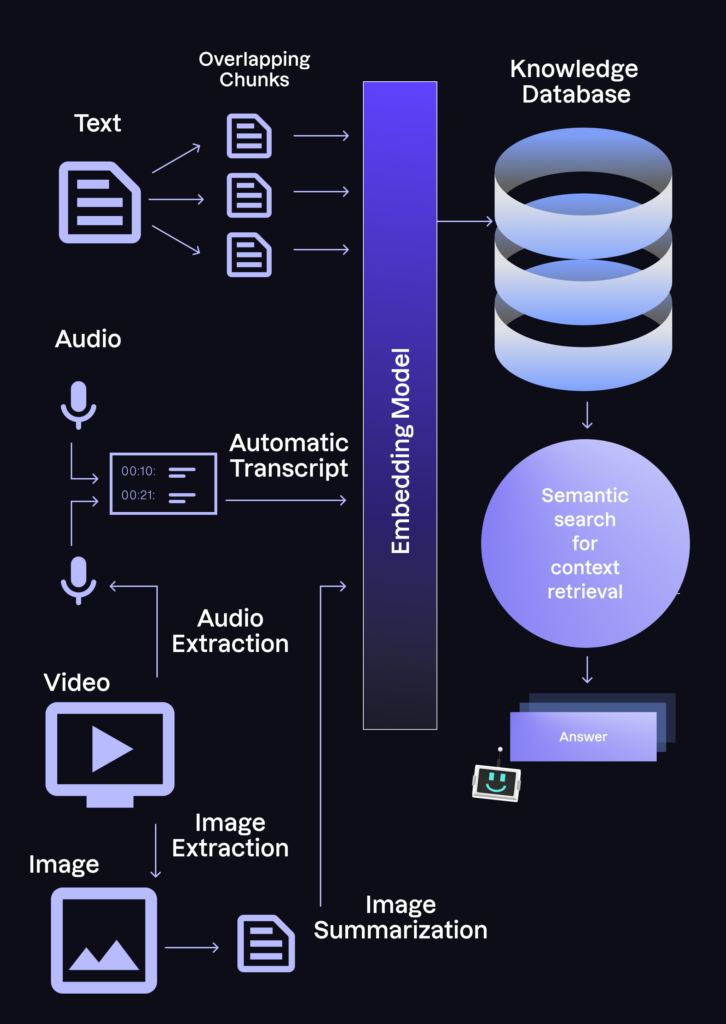
Initially, RAG systems were designed to operate solely on textual data. Whether it be Word documents, PDFs, web pages from an internal wiki, or repositories of computer code, any data source mappable to text sufficed for constructing a RAG system. This is primarily due to the fact that the knowledge database utilized in these systems is constructed with embeddings automatically extracted by large language models from small text fragments. In particular, every new text corpora needs to be dissected into (slightly) overlapping text chunks, passed through the embedding model to acquire its semantic, vector-based representations, and then stored in the vector database used for semantic search and context extraction.
However, with the advent of multimodal foundational models, the opportunities are limitless. Now, we can process images, videos, and even sound to augment the data sources available to a RAG system. Consider the possibilities: you can now inquire about the specific segment of a recorded conference call where you discussed a particular topic with a customer, or instantly access images with a very specific characteristic, simply by typing into a search box.
Achieving this capability remains a challenge, though. It requires a proficient team that can generate a custom data processing layer capable of transforming data in multiple formats into elements compatible with the embeddings model. For example, at Arionkoder, we harness GPT-4 Vision to extract textual insights from screenshots of our demos or slides. Then, these in-depth summaries can be processed by the LLMs just as we do with the text chunks. Similarly, for video and audio, we extract transcripts with timestamps using off-the-shelf tools, enabling us to pose questions and receive immediate references to specific moments with the appropriate prompting and storage.
The potential applications for RAG systems are therefore boundless. We can now develop data co-pilots for digestion in almost any context: retrieving information about contracts, scientific papers, electronic health records, personal family videos, and photographs. All that’s required is the ability to process and summarize their content, and the possibilities become limitless.
As data continues to proliferate, the importance of robust RAG implementations becomes increasingly evident. For organizations seeking to harness the power of this transformative technology, Arionkoder stands ready to provide tailored solutions and expertise in RAG implementation. Reach out to us today at hello@arionkoder.com to unlock the full potential of your data landscape.