This post continues part 2 of our series “The Future of Healthcare is Here: Arionkoder’s Comprehensive Guide to AI in Medicine”. Find part one here, and our previous post on the data dimension here. Stay tuned for more insights and practical tips from Arionkoder’s experts on harnessing the power of artificial intelligence in healthcare.
The daily routine of a clinician is filled with constant evaluations and judgment calls, and the success of these can be greatly influenced by various factors such as the availability and completeness of information, the tools used to analyze and integrate that information, and the clinician’s background knowledge and experience.
The stakes are high in healthcare, as the outcomes of these decisions can greatly impact patients’ health and quality of life. With the advent of AI, there is an opportunity to develop more efficient decision-making processes and clinical support systems that can aid medical professionals in making better-informed decisions in a shorter amount of time.
However, developing such systems requires a thorough understanding of the current decision-making process, the expected solution, and how its success will be measured. At Arionkoder, our strategy when studying the problem dimension is to decompose its definition into a series of processes or use-cases. Once we identify them, we clarify to our clients the data needed for crafting their corresponding solutions, and delineate with them a development plan (that we will cover in the upcoming post!).
While the variety of projects and challenges in automating healthcare with machine learning can be complex and overlapping, the following are in our opinion the most frequently encountered problems in AI for healthcare. Let’s delve into how they vary depending on the input data, and which are the specific data needs for each of them.
Automated diagnosis
Automated diagnosis using AI can greatly improve and accelerate the process of identifying diseases, screening for them, or evaluating their severity. In traditional medical practice, clinicians make a diagnosis relying on multiple sources of patient-specific data, including symptoms, medical images, and lab results. However, with the advent of precision medicine and advancements in medical imaging, the amount of available information has become so big that it has turned overwhelming and difficult to manually process.
Machine learning algorithms can automate this task by detecting disease related patterns in large storages of multimodal data. The input to these models can come in various forms, including electronic health records, lab results, medical images, etc, all of which can be used to make an automated diagnosis.
Depending on the specific task, the output of an automated diagnosis system can take various forms. For example, an AI model trained to detect referable diabetic retinopathy cases from color fundus photographs can predict a binary warning label indicating if the patient is at risk for blindness. Other algorithms trained to stratify the disease grade may output an ordinal variable, with a score of 0 indicating a healthy status and higher values indicating an increased level of the disease. In some cases, AI models can predict the presence of multiple diseases at once, which is useful for screening target populations and making individualized decisions.
To train these algorithms, it is necessary to access a database with multiple patient samples annotated as diseased or not, or reflecting the grade of the disease. The AI model will learn to predict this variable on new input samples from unknown patients by minimizing the errors committed on this training data set.
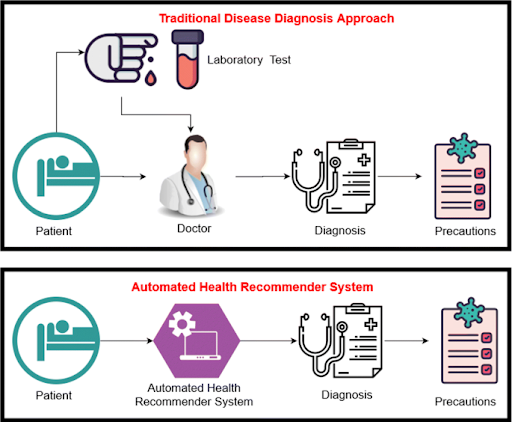
Predictive modeling
AI based predictive modeling can significantly enhance clinical decision-making by bringing valuable predictions to healthcare providers about patients’ future outcomes and characteristics. Without it, healthcare professionals often rely on their own experience and intuition, which can lead to inconsistencies and errors.
One of the key advantages of predictive modeling is its ability to identify patients at high risk for certain outcomes, such as mortality or disease progression. Early identification of these patients enables clinicians and medical institutions to take appropriate measures to avoid undesired results and achieve specific goals. Additionally, predictive modeling can be used to forecast the risk of developing a certain condition, or to preview the effectiveness of different treatment options for individual patients (see the “Personalized treatment…” section), assisting providers to make more informed decisions about therapeutic plans.
The specific data requirements for these applications can vary depending on the task and input data. When the objective is to predict patient mortality or disease progression, for example, the outcome can be obtained from patient demographic information, his/her medical history, lab results, medical images or clinical signals. Similarly to what occurs for automated diagnoses, training these models requires having access to a large database of past patient samples, but this time each of them have to include the outcomes that are expected to predict in test time. Hence, the model will in this case be trained to predict with a minimum error these target values. When deployed, this past knowledge will be exploited by the algorithm to forecast those targets on new input samples for which this value is still unknown.
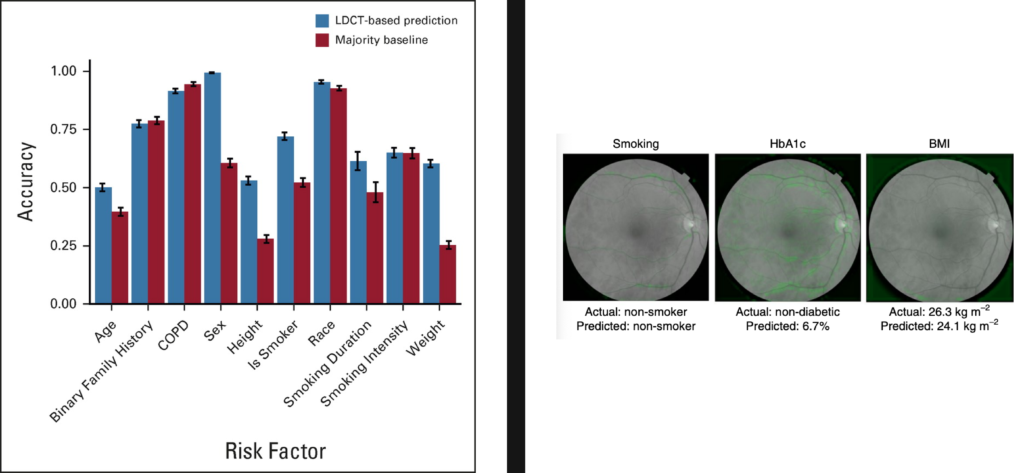
Medical image analysis
Medical image analysis is a critical task for radiologists, who are responsible for interpreting and identifying any abnormalities or conditions in medical scans. While this duty is already well-established in a conventional clinical pipeline, it is complex, time-consuming and overall challenging. Some lesions may be too subtle to recognize with the naked eye, and different radiologists can disagree in their opinions for the same given image. Furthermore, a radiologist may even contradict him/herself when analyzing the same image twice.
AI systems based on computer vision technology have the potential to empower radiologists and help them analyze a larger number of images in a more efficient, consistent, and accurate manner.
The input data to these algorithms is a medical image, of course, although it can vary depending on the task: it can be a 2D image, such as an X-ray scan, a fundus photograph, a mammogram, or an ultrasound image; it can also be a higher dimensional scan, such as a 3D CT or an MRI; or it might be even a video sequence, such as in the case of angiographies.
The outcomes also depend on the target application. For example, medical images can be used as input for automated diagnosis, in which case the output of the algorithm will be the same as detailed in the previous section. These scans can also be used to quantify the status of the patient, e.g. by counting the amount of lesions due to a certain condition or their location. Furthermore, AI models can be used to improve patient monitoring and measure their response to a specific treatment, e.g. by automatically detecting and measuring the size of a tumor at multiple visits. Radiologists can also use AI for registering images taken at different time points or belonging to different modalities, to compare one another and extract in-depth insights for decision making.
To build and evaluate these solutions, it is crucial to have access to high-quality, relevant data. These needs also vary depending on the target applications. For example, for automated diagnosis, it is necessary to have access to a large, diverse number of labeled images of healthy individuals and of patients suffering from the target diseases. When the task is to detect and quantify lesions, it is necessary to have annotated images with delineated lesions. If the objective is to register multimodal scans, having a series of aligned examples is crucial to train the algorithms to automate the task and to evaluate if the obtained results are accurate.
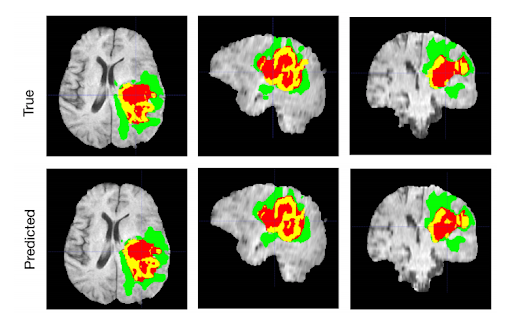
Supporting clinical trials
Conducting clinical trials is a vital step in the development of new drugs and treatments for a given disease. The process involves selecting a group of candidates based on various factors such as demographics and health conditions, administering the drug or treatment to these candidates, and comparing the results to a placebo or other baseline treatments. The data collected during the trial is then analyzed to determine the effectiveness of the new therapy.
However, analyzing such a vast amount of data can be time-consuming and challenging to perform manually. AI offers an opportunity to automate several steps in this process, such as using machine learning models for medical image analysis to measure variations in lesion size or number to the administration or not of the tested drug, thus bypassing the need for manual expert analysis.
AI can also be used to improve the organization and management of clinical trials. Predictive models can be used to optimize logistics, such as identifying potential candidates for the trial, forecasting the necessary resources, and predicting the likely outcomes of the trial. This can help to increase the efficiency and effectiveness of clinical trials, ultimately leading to faster and more accurate conclusions about the safety and efficacy of new treatments.
Depending on the desired outcome, we might need to use models for medical image analysis, predictive modeling, etc. Hence, this is one of those overlapping problems that we previously mentioned, where the final solution is achieved by taking tools from some of the other problems we described before.
Personalized treatment and computer-aided interventions
Clinicians use a combination of patient data, medical history, and expert knowledge to decide the best course of treatment for a patient. This process can be time-consuming and prone to inconsistencies and errors, as it relies on the clinician’s subjective interpretation of the data.
AI can be used to automate and optimize this process, by analyzing large amounts of patient data and finding which treatment tracks are the most appropriate to ensure successful therapeutic results. Hence, given input information about the patient such as a medical image, his/her electronic health record, or even text describing their overall condition, these models can bring clinical support by choosing a drug or specific intervention, based on previous experience. You can think of this as a specific type of predictive model, trained using past cases, the treatments that were individually adopted for each of them, and their final outcomes. The model will learn the relationship between patients’ features, the treatment and its results, and avoid those that can fail or be dangerous for the individual.
These algorithms are used e.g. to aid in personalizing the selection of the most appropriate intraocular lens to implant, to avoid post-operative complications such as cataracts or glaucoma. The inputs are in this case patient specific demographics, characteristics of his/her visual condition, and measurements taken from medical images and using dedicated devices.
Computer-aided intervention, on the other hand, is another promising field for applying AI. These tools are increasingly used by surgeons to complement their skills and assist them when performing complex procedures. By analyzing patient data and providing real-time guidance, these AI-driven solutions can help to reduce the risks and improve the outcomes. In colorectal interventions, for instance, computer vision models can be used to automatically detect polyps that need to be removed by the clinician, directly from the interventional footage. Similarly, laparoscopic surgeries can be assisted by AI algorithms that detect the location of tools and organs and prevent accidents or confusion.
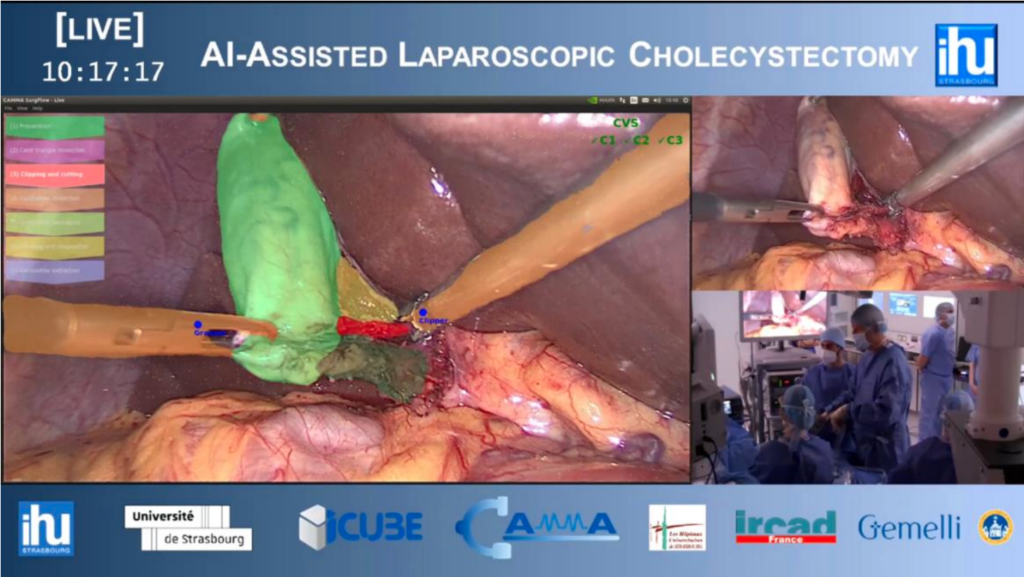
Medical record management
Medical record management using AI can improve the efficiency and accuracy of the process of collecting and recording patient information.
When a patient visits a doctor or enters a hospital, clinicians often have to manually input information into the electronic health record. This process can be tedious and take a long time, as the professional spends many minutes typing text into the system.
AI can be applied to automate the process of collecting patient information. This can reduce the time and effort required to manually enter data, and increase the accuracy of the information recorded. Additionally, AI can be used to standardize the text produced by multiple experts, who may use different clinical jargon, to a uniform standard that can be easily understood by any new specialist. Furthermore, machine learning can automatically process and transcribe handwritten notes, such as prescriptions, into text, which can improve the accuracy and efficiency of the medical record management process.
Drug development and discovery
Drug discovery and development is a long and complex process that involves identifying new compounds, testing them for efficacy and safety, and bringing them to market. AI can be used to assist in this process by analyzing large amounts of data and identifying potential drug candidates.
One way AI can be used in drug discovery is through virtual screening. This involves using computer simulations to identify compounds that are likely to interact with a specific target protein, and thus have the potential to be developed into drugs. By analyzing large amounts of data on chemical compounds and their interactions with biological systems, AI models can predict which compounds are most likely to be effective in treating a specific disease.
Another application of AI in drug development is through the optimization of drug design. This involves using AI algorithms to predict the physical and chemical properties of a compound, such as solubility and stability, and to design molecules that have the optimal properties for development into a drug.
AI can also be used in the preclinical development phase, in which potential drug candidates are tested in laboratory experiments and animal models to determine their efficacy and safety. By analyzing data from these experiments, machine learning models can predict the potential side effects of a drug and identify potential safety risks.
Overall, we have seen that capturing requirements for AI projects in medicine requires comprehensively analyzing the original problem from both a data and a use-case perspective. By isolating common, prone-to-automate tasks and identifying their specific data needs, our skilled team of experts is able to properly identify the opportunities offered by our clients’ databases and come up with a plan to achieve a successful solution.
Stay tuned for our next article explaining how we entwine the data and problem dimensions to choose the most convenient AI tools for each challenge. And reach out to us to discover how we can help you implement these game-changing solutions!