By José Ignacio Orlando, PhD -Subject Matter Expert in ML/AI @ Arionkoder- and Nicolás Moreira -Head of Engineering @ Arionkoder-.
Image classification is one of the fundamental tasks in computer vision, helping automate processes, reduce costs and improve efficiency in many domains. In this post, we provide you with a comprehensive guide on how to take advantage of this technology for your business.
As we have discussed in our previous post, the goal of computer vision is to allow machines to understand images and extract value from them. In this context, image classification is one of the fundamental tasks in the field, seeking to assign a class to a given image. While this might sound simple to do for us as humans, making a computer decide in which “box” to place an image in is actually quite challenging. Hence, for many years many researchers were stuck on this problem, trying to hand-craft domain-specific algorithms that were then not suitable for generalization to other problems. But since the resurrection of convolutional neural networks back in 2012, solutions to image classification have experienced a (seriously, fast) boost, reaching accuracies that are either comparable or superior to those obtained by human observers.
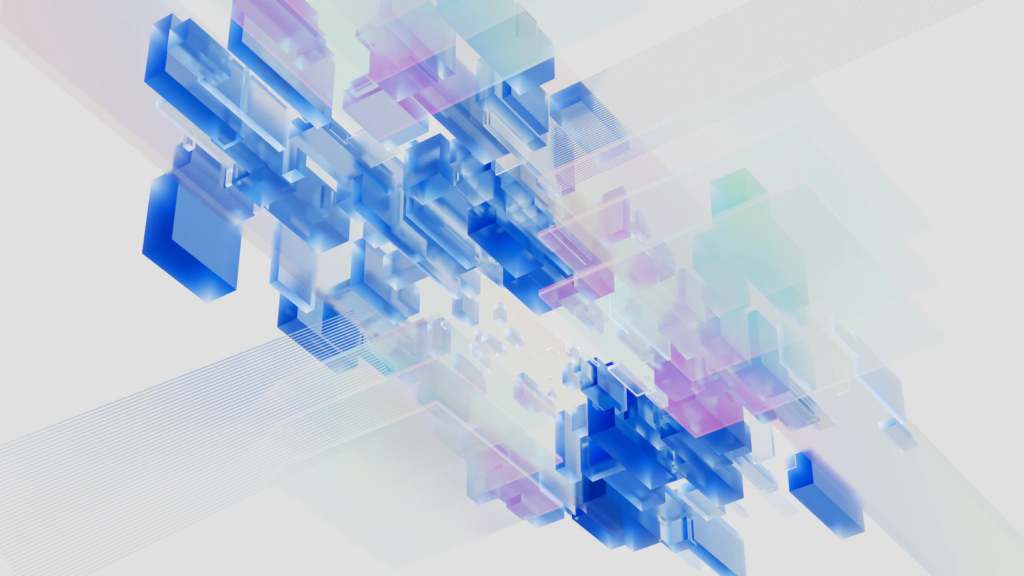
But let’s step back and talk a bit more about the problem itself. We previously said that we want to assign a class to an input image. By images we not only refer to pictures taken with standard cameras but to any graphical resource, including a scanned version of a document, satellite imagery or even medical scans. The class, on the other hand, is a categorical variable with meaning, such as “dog”, “cat”, “a number 9”, “a field of grain”, “cancer”, etc.
Such a general framework can be extensively applied in many domains. Here we cover a few examples, but don’t hesitate to contact us if you want to know how to use it in your own business:
- Optical character recognition (OCR). This technology can automatically analyze -parse- words and numbers from images by classifying each observed character of a scanned document into its associated character or digit. At Arionkoder, we are using OCR as part of OncoRx to automatically mine relevant information from molecular test reports and help clinicians to combat cancer.
- Automated diagnosis from medical images. An image classifier can immediately determine if an image shows signs of a certain disease. This can help radiologists produce reports efficiently, sorting risk cases in advance to be analyzed first, or detecting highly risky pathologies before having an expert called in. At Arionkoder we have AI consultants with a proficient academic background in medical imaging that can help your business and find new opportunities in this field.
- Quality control and automated inspection. Image classification models can be used to detect abnormalities in products based on their pictures, replacing expensive visual inspection and accelerating throughput.
- Face recognition and security surveillance. Either from real-time footage from security cameras or by analyzing past video imagery, image classification models can be used to identify individuals based on their facial features at a lower cost and with high precision.
By now we’ve already presented evidence to support the value of automating image classification tasks. But… How is it actually done? Stay tuned for our new post next week covering how we handle these problems at Arionkoder. In the meantime, be sure to reach out to us and discover everything we can accomplish working together!